Scientific Findings
Get insights from Lirio behavioral science and AI experts on how to move people toward healthy behaviors and actions that drive positive outcomes.
BeSci Briefs
Our BeSci briefs cover the behavioral science solutions Lirio uses to optimize the content, channel, and timing of behavioral interventions and the behavioral biases that inform them.
Anticipated Regret: Why Pondering an Unpleasant Future Can Change Your Behavior Now
It’s New Year’s Day, 2022, and you just woke up. You pour yourself a cup of coffee and settle into your favorite spot on the couch. As you gaze out the window, you feel a sense of calm and hope. There’s a brand-new year ahead of you. As you think about what lies...
Curiosity – Lirio Bias Brief
Lirio delivers results by drawing insights from hundreds of behavioral biases and theories. Our Bias Brief series walks through specific biases one by one, each thoughtfully selected from our long list of insights. Read on for a brief rundown on the chosen bias,...
Availability Bias – Lirio Bias Brief
Availability Bias is a mental shortcut that leads people to assume that things they can more easily recall are more likely to occur. As a result, people tend to weigh their judgments toward more recent information.
3-minute read
Temporal Landmark Effect – Lirio Bias Brief
Dates that stand out as being more meaningful—such as the start of a new week or financial quarter, a birthday, or a holiday— signal the start of a new, distinct time period. These “temporal landmarks” make people more motivated to pursue their goals because specific dates make it easier for them to mentally separate their past imperfections and failures from their future self.
4-minute read
Educational Sessions
Our team has led informative discussions and presentations about the power of combining behavioral science and AI to create behavior change.
Leveraging Behavioral Design to Increase COVID-19 Vaccinations
Greg Stielstra, host of The Behavior Change Podcast, welcomes guests Sarah Delaney and Ashley West to discuss the behavioral design report that the pair helped create to increase COVID-19 vaccinations. Subscribe to The Behavior Change Podcast The thoughts and...
How to Leverage the Complexities of Memory to Drive Behavior Change
The Behavior Change Podcast by Lirio explores the various ways humans can leverage behavioral science to personalize our messaging, engage our audience, and drive better behavior at scale. Subscribe to The Behavior Change PodcastTranscript Greg Stielstra: Hello, and...
Inspiring Better Habits: The Science Behind Making Commitments
The Behavior Change Podcast by Lirio explores the various ways humans can leverage behavioral science to personalize our messaging, engage our audience, and drive better behavior at scale. Subscribe to The Behavior Change Podcast Transcript Greg Stilestra:Hello and...
Solving From the Outside In: the Art of UX Design
The Behavior Change Podcast by Lirio explores the various ways humans can leverage behavioral science to personalize our messaging, engage our audience, and drive better behavior at scale. Host: Greg Stielstra (GS), Senior Director of Behavioral Science at Lirio...
Academic Research
Our team has developed and presented a range of academic research pieces across the fields of behavioral science and AI.
Artificial Intelligence
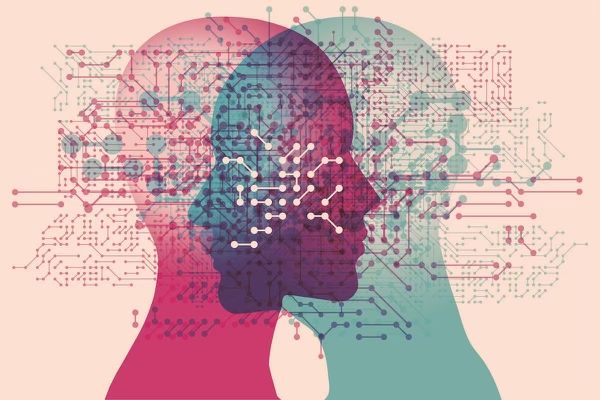
Lirio Research: A Novel Policy Comparison Metric for Reinforcement Learning
Lirio’s Behavioral Reinforcement Learning Lab (BReLL) recently published a paper describing a new approach, the Limited Data Estimator, for comparing reinforcement learning policies using limited historical data.
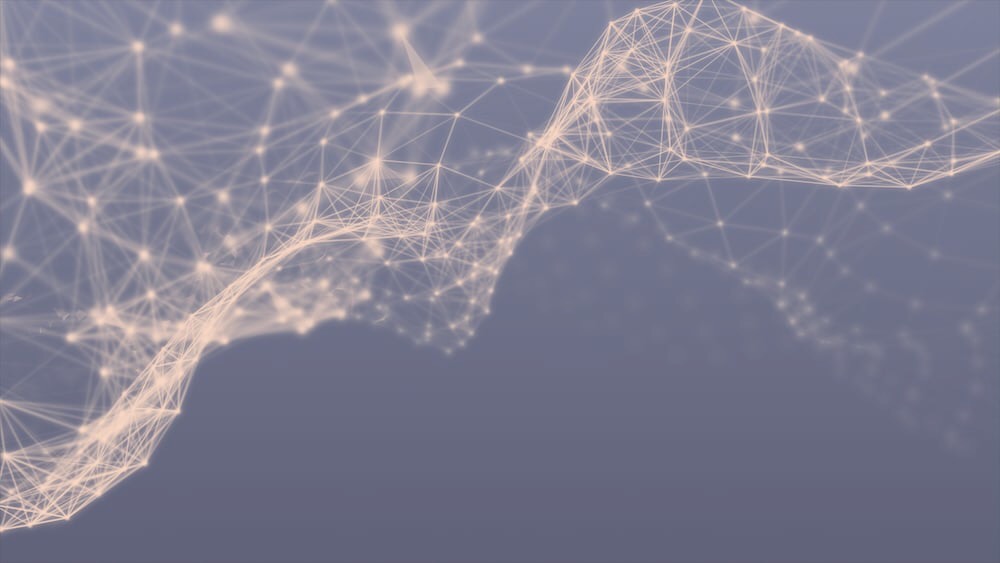
Lirio Research: Introducing A New Form of Machine Learning Optimization
Lirio’s AI Research team recently developed a novel adaptive stochastic gradient-free (ASGF) approach for solving some of the most difficult optimization challenges in machine learning. This innovative optimization algorithm, which is simple to implement and does not require careful fine-tuning, offers significant improvements when compared to existing state-of-the-art approaches.